AI predictive analytics often promises transformative insights but can sometimes feel more like science fiction than reality. Many of us eagerly embrace this technology, hoping for precise forecasts and improved decision-making. Yet, frustration mounts when outcomes fall short. Why do these powerful tools sometimes miss the mark? You might be surprised to learn that the failure often stems not from the technology itself but from overlooked factors in its application.
Legal predictive analytics, for example, can revolutionize decision-making processes but demands careful integration of multiple datasets. The same goes for predictive models in other fields. When AI fails, it usually signals that human oversight is needed. Understanding these pitfalls is essential to tap into the true potential of AI-driven insights.
Curious to know why AI predictive analytics can fail and how you can avoid common mistakes? Let’s dive deeper into this fascinating topic, where I’ll share practical tips and insights to turn AI challenges into opportunities for success.

Photo provided by Mikhail Nilov on Pexels
Inside the story
Understanding AI Predictive Analytics
When I think about AI predictive analytics, I’m fascinated by how it can anticipate future outcomes. This technology helps in making accurate forecasts by analyzing vast amounts of data. It’s like having a crystal ball, but powered by data. AI does this by spotting patterns in historical data, which allows it to predict what might happen next. It’s amazing how AI can transform raw numbers into meaningful predictions. However, it needs quality data to work effectively. Without good data, the predictions can go off track.
Common Pitfalls
Despite its potential, AI predictive analytics can sometimes fail. One big reason is the quality of data fed into the system. If the data is flawed, the predictions will be too. I’ve noticed that data inconsistencies and gaps often lead to unreliable predictions. Another issue is the misinterpretation of results. Sometimes, people see what they want to see rather than what’s actually there. This can lead to major mistakes in decision-making.
AI Predictive Analytics
Data Quality Issues
Data quality is crucial for AI Predictive Analytics. Poor quality data can produce inaccurate predictions. I can’t stress enough how important it is to ensure data is clean and consistent. When data is messy, the entire prediction model becomes unreliable. I’ve learned that properly managing data can help in generating more accurate predictive analytics outcomes. Good data leads to better insights and more reliable forecasts.
Misinterpretation of Results
Misinterpreting AI predictions can cause significant problems. When I analyze results, I make sure to understand what the data is truly saying. It’s easy to read too much into the numbers or overlook the details. I’ve found that having a clear understanding of the context and limits of AI predictions helps in making informed decisions. It’s about interpreting the results accurately and not jumping to conclusions.
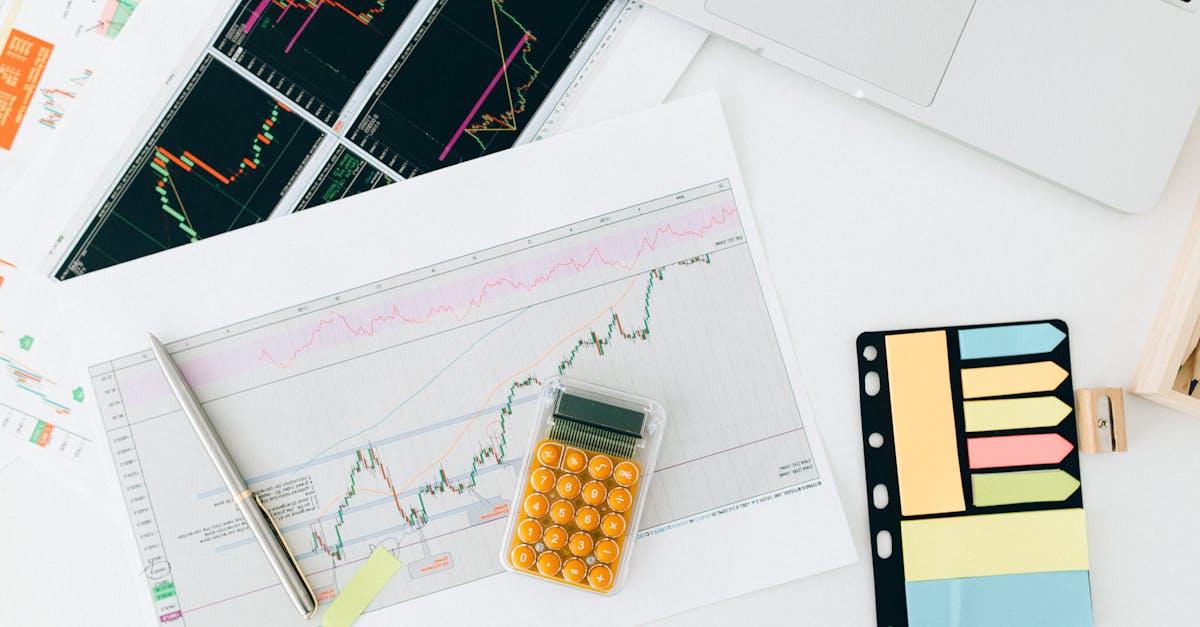
Photo provided by Nataliya Vaitkevich on Pexels
Legal Predictive Analytics Challenges
AI in Legal Decision-Making
AI is becoming crucial in legal decision-making. I’ve seen how it helps predict legal outcomes by analyzing past cases. However, its influence is only as good as the data it analyzes. If the data lacks depth, the predictions can be off the mark. Legal predictive analytics requires carefully curated data to be truly effective.
AI-Based Legal Outcomes
The impact of AI on legal outcomes is profound. It can help foresee potential verdicts and even suggest possible arguments. But the reliance on AI must be balanced. I think it’s vital to ensure that AI complements human judgment, rather than replacing it. A good balance leads to better decision-making overall.
Improving Data-Driven Legal Insights
To improve data-driven legal insights, strategies must be in place to enhance data quality and interpretation. I recommend leveraging diverse datasets to gain a more holistic perspective. This helps in ensuring the insights are comprehensive and actionable. Legal professionals need to embrace these strategies to fully harness the power of AI in legal settings.
Wrap Up: Understanding and Improving AI’s Role
Reflecting on what we’ve discussed, it’s clear that the right approaches can unlock the true potential of predictive models. You’ll gain insights into trends and behaviors by using these models effectively. This will aid in making informed decisions that drive your goals forward. Mastery over these methods ensures that your efforts in data-driven fields become more accurate and reliable.
Start by reviewing your existing tools and identify any gaps or areas for improvement. Consider integrating more diverse data sources to broaden the scope of your analysis. Explore learning resources or workshops to enhance your understanding and skills further. This active approach will help uncover insights that were previously overlooked.
Now, take the first step toward optimizing your systems. Commit to exploring at least one new method or tool this week. Your proactive steps today will build a stronger, more effective framework for tomorrow’s challenges. Ready to transform your approach? Dive in and start today!